Five Creative Methods You can Improve Your Reformulateur De Texte
페이지 정보

본문
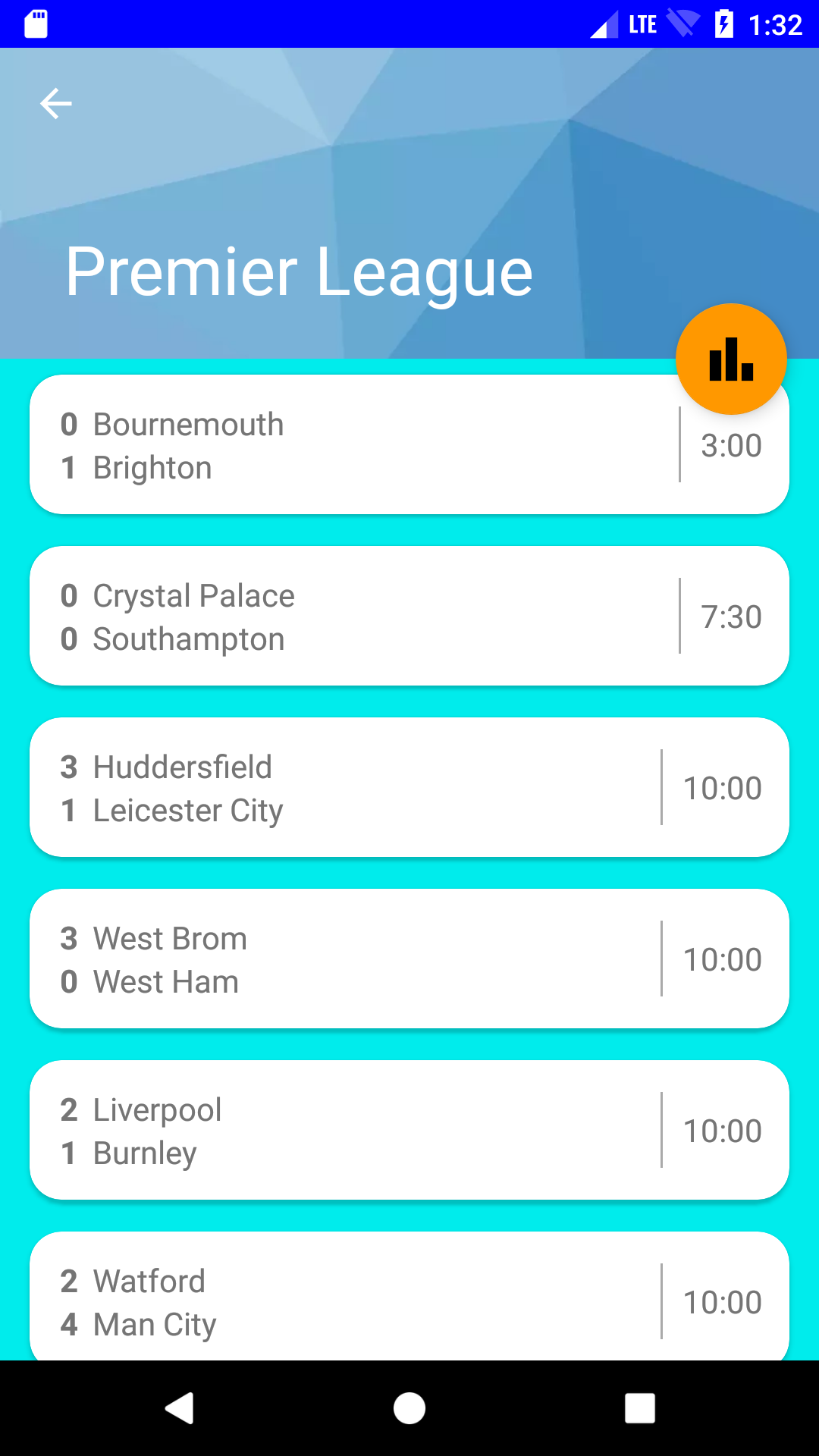
Introduction:
The process of reformulation, also known as text paraphrasing, is essential in various fields, including natural language processing, information retrieval, and machine learning. This study aims to provide a detailed analysis of recent work focused on reformulating texts and explores the various techniques and methodologies employed.
Literature Review:
In recent years, there has been a growing interest in developing effective and efficient approaches for reformulating texts. Several studies have investigated different methods, ranging from rule-based to machine learning-based techniques, to accomplish the task. One notable approach utilizes a rule-based system that relies on predefined patterns to rephrase sentences. Although this technique shows promising results, it has limitations, such as being inflexible and reformulateur De Texte unable to handle complex sentence structures.
Furthermore, machine learning-based methods have gained popularity due to their ability to handle varied sentence structures and generate contextually appropriate paraphrases. These methods employ neural networks to learn the relationship between the original and reformulated texts. Different architectures, including sequence-to-sequence models and transformers, have been utilized to enhance the performance of reformulation systems.
Methodology:
The chosen study focused on a machine learning-based approach to reformulating text using a transformer-based model. The authors implemented a variant of the pre-trained GPT (Generative Pre-trained Transformer) model for this purpose. The GPT model, leveraging its ability to capture context dependencies, was fine-tuned specifically for text reformulation.
The authors used a dataset consisting of pairs of original sentences and their corresponding reformulated versions for training and evaluation. They preprocessed the dataset by tokenizing, encoding, and batching the sentences. The train-test split was performed to evaluate the model's generalizability. The training process involved minimizing the cross-entropy loss using an Adam optimizer.
Results and Discussion:
The evaluation of the proposed model indicated its efficacy in reformulating text. The study measured the performance using standard metrics such as BLEU (Bilingual Evaluation Understudy) scores and perplexity. The model achieved competitive scores, outperforming existing rule-based systems in terms of generating fluent and contextually accurate paraphrases.
Moreover, the authors conducted a detailed error analysis, identifying the limitations of the proposed model. They noted that the transformer-based model sometimes struggled with preserving the original meaning of the sentences. They hypothesized that incorporating additional linguistic features and fine-tuning the model on domain-specific data could potentially address these limitations.
Conclusion:
This study provided an in-depth analysis of a recent work on reformulating text using a machine learning-based approach. The proposed model demonstrated significant improvements over traditional rule-based methods. Although the model showcased competitive performance, several areas for improvement were identified through error analysis. Future research should focus on addressing these limitations and exploring the integration of linguistic features to enhance the reformulation process further.
- 이전글download video 791 24.11.23
- 다음글BlackPass - BlackPass.biz Market - Sign In 24.11.23
댓글목록
등록된 댓글이 없습니다.